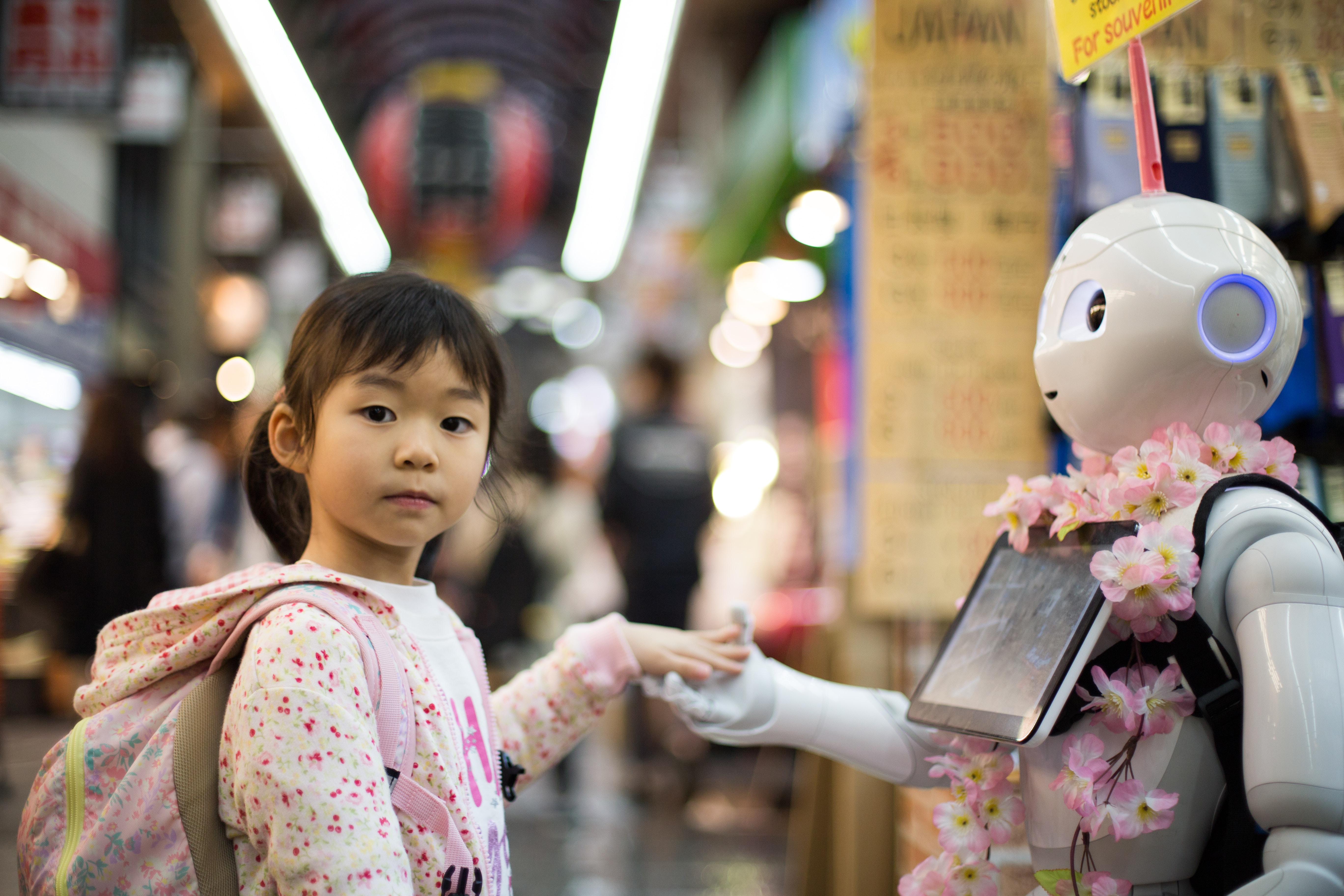
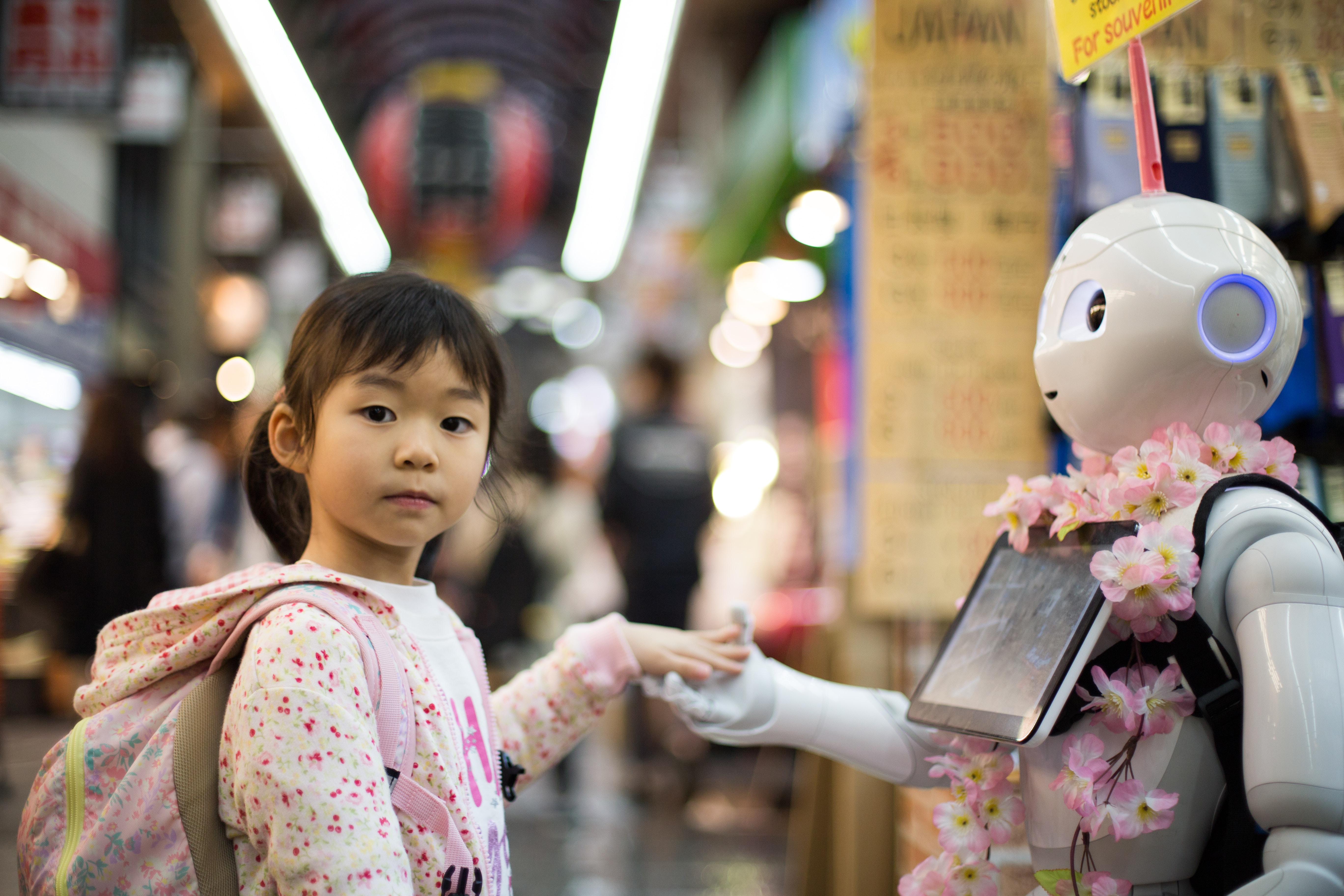
Part truth, part sci-fi, the notion of rogue artificial intelligence is gaining a lot of attention — for good reason.
As the creators of AI, humans hold responsibility over sentient machines in a manner similar to parental duties. But as AI matures into programs that can spawn and train themselves, things become less explainable by humans and more autonomous for machines. AI’s complexities have led to the call for more diversity of backgrounds and soft skills in the field in hopes of curbing bias and an all-out derailment of AI through more thoughtful training.
All of which suggests an intriguing question: Are parents uniquely equipped to train AI, and could a parenting method better train AI to abstract and adapt in today’s rapidly advancing business world?
Recent developments have catapulted AI’s advancements, enabling AI’s degree of cognition for the Gestalt test, realistic text generators and accurate medical diagnoses. And for every type of AI, there’s an engineer behind the scenes, programming the software to recognize patterns in massive data sets to achieve a particular objective.
To that end, a parentlike approach is already in use with reinforcement learning, which guides an AI’s initial development so that it can learn quickly from its mistakes and self-correct accordingly. At the same time, developments in neural networking for AI have imbued machines with even more human-like qualities, begging the question: Will AI soon be able to think abstractly enough to generalize beyond niche use cases for broader business applications?
To gain a better understanding of parental-style training methods in the dizzying world of AI, I recently spoke with James Kobielus, lead analyst for data science at SiliconANGLE’s sister market research firm Wikibon. For more than a decade, Kobielus has been closely analyzing the depths of AI, from computing infrastructure to ethical frameworks. It will take a village of skill sets and AI training methods, spanning supervised and unsupervised models, to prepare AI for the grownup tasks of modern business, according to Kobielus. The following has been condensed for clarity.
Q: How could parental experience contribute to the soft skills helpful in AI training?
A: Any sentient creature, from an octopus to a tree, engages in some degree of learning. They have to, as it were, adapt to different environments. We’ve evolved to learn, and that’s why we’re still here. So how does a person learn? There’s nature, and there’s nurture.
Let’s talk about nature. As an invention of man, AI is refined and adapted to various tasks. When we’re talking about learning in the AI context, it’s able to adapt its behavior to changes in the environment, meet the challenges it faces in that environment, and has some degree of success in reaching its outcomes. In the past 10 years, AI has shifted almost entirely from rule-based systems to what we now call machine learning.
In parenting, to some degree, a parent doesn’t need to give their child a lot of things. Babies are born with cognitive skills built into their wiring. Learning isn’t training in the sense that there’s some specific task to do to ensure unsupervised learning. But for AI, supervised learning is building a model to see how it can process data in a way to predict what it will see next based on how it’s been trained on historic data. Examples include the prediction of age, race, gender or facial recognition.
In the context of parenting and AI, building predictive models reasonably well is a soft skill, as you have to understand what causes what. For reinforcement learning, you’ve got to understand the task, and you want to make sure people aren’t being harmed and objects aren’t being damaged. There has to be extensive simulations in reinforcement learning — with autonomous cars, for example. It’s the same for parenting; there’s lots and lots and lots of rules. Parents give a long or short reach based on the risks of the environment. Kids don’t learn all on their own, but they do have innate limiters.
Q: How could reinforcement learning help curb bias in AI models?
A: When it comes to bias in AI, it’s defined as a set of outcomes you want to avoid. Every AI has a bias toward the task for which it’s trained. When we talk in a broad sense of the perfect AI model, it comes down to the soft skills of the AI builders understanding the task to be achieved. There’s lots of variables, such as protected attributes, when building AI for home loan approvals, as an example. While there may be valid predictors in a loan, if they’re baked into the AI model, it could effectively unfairly bias entire groups of people that didn’t have historic advantages like wealthy parents or private schooling.
How AI can curb bias is: Focus on the data. It reflects the bias in society at large so the AI can be designed against unwanted bias. The AI model must be tested for potential bias, which can be done by a human workforce to evaluate those outliers identified by the AI.
For reinforcement learning to avoid bias, it’s actually not something I’ve come across yet, so let’s explore it. If an AI is programmed to avoid the steps that might be correlated with unfair discrimination, it’s possible to use reinforcement learning to train an AI model to take steps to avoid overt bias.
Q: What can machines do better than toddlers? What can toddlers do better than machines?
A: Let’s just say humans, because toddlers are humans. Well, humans aren’t programmed — we’re not machines. At no point has someone written code to be directly inserted into my brain. I instead take information and assess it. That’s how we learn.
AI must achieve logic from humans, who can program in hard logic and soft logic. And logic is becoming increasingly statistical. That’s what the AI revolution is all about. Machines run 24/7, don’t sleep or burn out. Machines can process far more data than humans and can keep an updated, precise data log, while I can barely recall what I said two seconds ago.
For AI, what’s so amazing is the chipset. The industry is moving toward AI-optimized chipsets, graphics processing units and Tensor Core processing. The logic that drives machines of all sorts, especially edge devices like my smartphone and Alexa sitting on my desk, is able to learn from its environment with amazing versatility to engage with humans.
But what humans can do better than machines is analogize. We can compare what we saw before to what we’re seeing now. Analogies are the foundation of human intelligence, and AI is now being programmed to do analogies under supervised learning with statistical representations.
You could even look at AI as increasing the refinement of statistical analysis for humans. It’s taking all of our senses — vision, auditory, et cetera — to build and refine statistical analysis. What machines can do is rapid, across vast troves of data possibly invisible to humans. We have intuition and innate skills. Machines are symbiotic with us, pumping our own intuition with data.
Q: What advancements have you seen or do you anticipate that could help AI models develop more abstract thinking to generalize acquired knowledge across differentiated use cases?
A: There’s no such thing as a generalized AI to go from one task to another. When people talk about AI in a generalized way, they tend to gloss over the tasks AI are called on to do. AI exists to find correlations in data, whether those are causal or statistical. Most AI is designed to do categorization and recognition tasks, such as voice recognition or anomaly detection. When you look at the broad tasks of AI, much of what I described in these examples are done through supervised learning, which minimizes the loss function — the difference between data presented and the statistical function predicting the next thing.
When you talk about reinforcement learning, it’s a different beast. You write a statistical model, played out through trial and error. AI models are able to, based on the next data set the algorithm sees, continually rejigger the underlying algorithm to identify if a particular action gets the AI closer to its cumulative reward function — the end goal.
Robotics is the classic core of reinforcement learning, where you try all possible paths or actions within some space or environment. For example, a robot walking over and picking up a block and putting it on a shelf. If 99% of actions a robot takes don’t advance it toward its goal, the AI will rework its algorithms to delete those erroneous paths. In parenting, trial and error is inherent in us all. If you walk off the ledge of a 10-foot drop, you’re going to hurt yourself and avoid that outcome in the future.
Q: Where does transfer learning fit in?
A: Now there is transfer learning, which refers to a growing range of techniques to take statistical knowledge built up in prior AIs — for example, a computer vision model for recognition of humans that’s fast and accurate being applied to the new task of recognizing other primates, or even adapting that model to recognize individual cats and dogs. This approach is able to repurpose models of all sorts, with adjacent use cases.
Another example is natural language processing. It can take sentiment analysis built on English natural language AI and apply it to other English-speaking dialects. This involves taking statistical analysis in terms of the neural network structure, taking the feature set in terms of the predictors used for the original model, applied to similar but not identical scenarios.
Transfer learning is being used a lot in gaming, enabling games to learn from each other so that, based on how people play one type of game, that data and AI built for it can be repurposed to another game, automatically learn to a degree, to play another game with a high degree of effectiveness. We also see transfer learning in robotics — a robot can learn to walk in one environment, and that knowledge can be transferred to a robot learning to crawl in a similar environment.
Q: Is there a business incentive to generalize AI systems, or is it still more cost effective to train specific AIs for specific tasks?
A: It’s more cost-effective to train AI for specific tasks. You can take an existing investment in AI and, without too much rework, suit it to do other things you might find more useful. There’s only an incentive if the cost isn’t too excessive. There’s not a lot of atificial general intelligence being done in the real world. Where AGI is thriving is in science fiction, and AGI is nowhere near prime time. AGI is very futuristic, the kind of thing you simulate in Hollywood more than in the real world.
THANK YOU