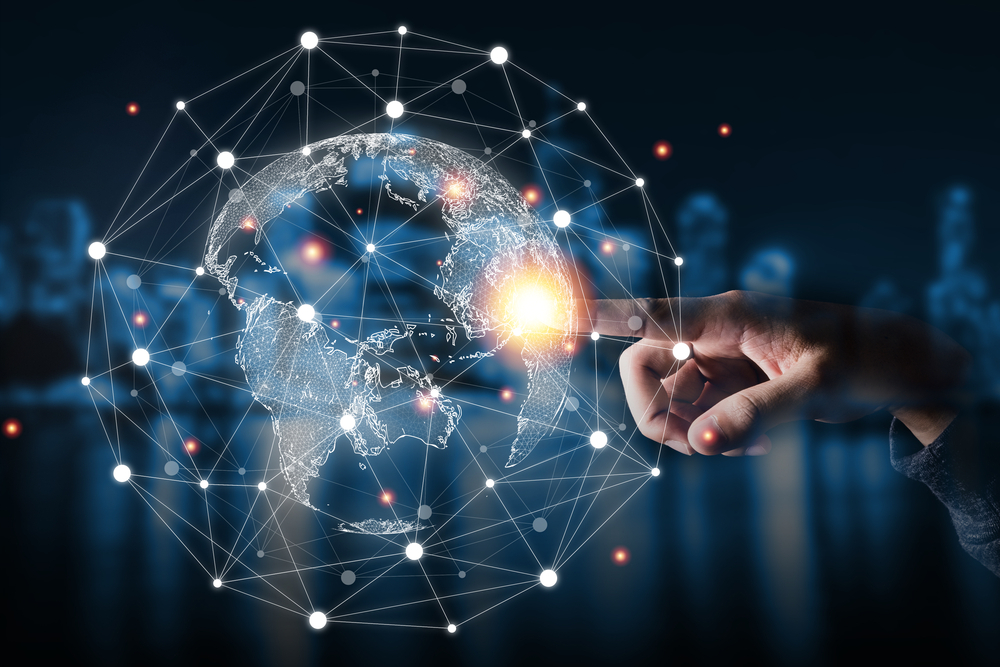
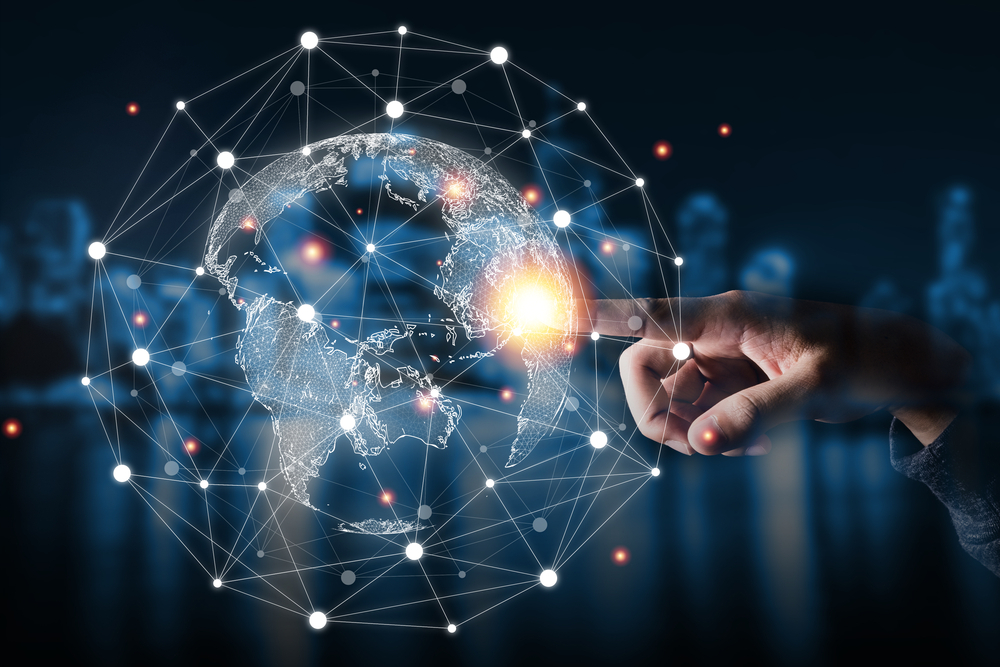
David Linthicum is enjoying a well-earned “I told you so.”
Six years ago the chief cloud strategy officer at Deloitte Consulting LLP predicted that the then-fledgling edge computing market would create a significant growth opportunity for cloud computing giants. That went against a popular tide of opinion at the time that held that distributed edge computing would displace centralized clouds in the same way personal computers marginalized mainframes 30 years earlier. Andreessen Horowitz LP General Partner Peter Levine summed it up with a provocative statement that edge computing would “obviate cloud computing as we know it.”
Not even close. There’s no question that edge computing, which IBM Corp. defines as “a distributed computing framework that brings enterprise applications closer to data,” is architecturally the polar opposite of the centralized cloud. But the evolving edge is turning out to be more of an adjunct to cloud services than an alternative.
Deloitte’s Linthicum: “Edge computing drives cloud computing.” Photo: SiliconANGLE
That spells a big opportunity for infrastructure-as-a-service providers such as Amazon Web Services Inc., Microsoft Corp.’s Azure and Google Cloud Platform. Most researchers expect the edge market will grow more than 30% annually for the next several years. The AI boom is amplifying that trend by investing more intelligence in devices ranging from security cameras to autonomous vehicles. In many cases the processing-intensive training models that are needed to inform those use cases require supercomputer-class horsepower that is beyond the reach of all but the largest organizations.
Enter the cloud. International Data Corp. estimates that spending on AI-related software and infrastructure reached $450 billion in 2022 and will grow between 15% and 20% annually for the foreseeable future. It said cloud providers captured more than 47% of all AI software purchases in 2021, up from 39% in 2019.
“We’re likely to see the cloud providers dominate these AI-at-the-edge devices with development, deployment and operations,” Linthicum said in a written response to questions from SiliconANGLE. “AI at the edge will need configuration management, data management, security, governance and operations that best come from a central cloud.”
There are good reasons why some people doubted things would turn out this way — starting with latency. Edge use cases such as image recognition in self-driving cars and patient monitoring systems in hospitals require lightning-fast response time and some can’t tolerate the time needed to round-trip requests to a data center a thousand miles away.
AI applications at the edge also require powerful processors for inferencing or making predictions from novel data. This has sparked a renaissance in hardware around special-purpose AI chips, most of which are targeted at edge use cases. Gartner Inc. expects more than 55% of the data analysis done by deep neural networks will occur at the edge by 2025, up from less than 10% in 2021.
“Inference at the edge is particularly important when you think about industries outside of the tech space like construction, long-haul trucking, or manufacturing,” said Evan Welbourne, head of AI and data at Samsara Inc., maker of a cloud platform for “internet of things” devices. “We’re dealing with petabyte-scale data in the cloud, but it’s more than a thousand times that scale at the edge.”
But most edge use cases don’t require near-real-time responsiveness. “For adjusting the thermostat, connecting once an hour is enough and it’s cheaper and easier to do it in the data center,” said Gartner analyst Craig Lowery.
“Latency isn’t an issue in most applications,” said Mike Gualtieri, a principal analyst at Forrester Research Inc. “You can get a very reasonable tens of milliseconds of latency if the data center is within 100 or 200 miles. Autonomous driving demands extreme locality, but those aren’t the use cases that are driving mass deployment today.”
Cloud providers stand to get much of that business, and they’re aggressively investing in infrastructure and striking partnerships to cement their advantage. Factors such as latency and edge intelligence will continue to exclude the big cloud providers from some applications, but there is so much opportunity in other parts of the market that no one is losing sleep over them. Even latency-sensitive AI applications at the edge will still require frequent updates to their training models and cloud-based analytics.
Forresters Gualtieri: “Latency isn’t an issue in most applications.” Photo: Forrester Research
No one expects the edge to be a winner-take-all market. Forrester’s recent Future of Cloud report said multiple players will compete with cloud providers for business at the edge, including telecom carriers, content delivery network providers and specialized silicon makers.
“Cloud providers will try to both beat these challengers and join them with cloud-at-customer hardware, small data centers, industry clouds and cloud application services, all with AI-driven automation and management,” the authors wrote. “As IT generalists, cloud providers will focus on AI-based augmentation rather than replacement of equipment and processes.”
That’s exactly what’s happening. AWS has built a network of more than 450 globally dispersed points of presence for low-latency applications. Google LLC has 187 edge locations and counting. Microsoft has 192 points of presence. All three cloud providers are also striking deals with local telcos to bring their clouds closer to the edge.
The real strength of the hyperscalers is providing the massive computing power that AI model training demands. The rapid surge of interest in AI that the release of OpenAI LP’s ChatGPT chatbot late last year has been a windfall.
“The cloud offers tools that are first-rate from silicon all the way through AI toolchains, maximum database optionality, governance choices, identity access, availability of open-source tools and a rich ecosystem of partners,” wrote Dave Vellante, chief analyst of SiliconANGLE’s sister research firm Wikibon.
Foundation models, which are adaptable training models that use vast quantities of unlabeled data, are considered to be the future of AI, but their petabyte-scale volumes require supercomputer-like processing capacity.
“Training requires lots of data that needs to be pumped into the modeling system,” said Gartner’s Lowery. “The power it requires and the heat it generates means it’s not something you’re going to do in a closet.”
“Foundational models are so sophisticated at this point that leveraging anything other than the cloud is impractical,” said Matt Hobbs, Microsoft practice and U.S. alliance leader at PricewaterhouseCoopers LLP. “For most companies, AI starts with driving the model in the cloud, moving some part of that to the device and then making updates as necessary.”
PwC’s Hobbs: Foundation models are so large and sophisticated that “leveraging anything other than the cloud is impractical.” Photo: PwC
Customers of Hailo Technologies Ltd., the developer of an AI chipset for edge scenarios, use cloud training models to create signatures that are then transferred to smart devices for image processing. “All of the companies we work with are training in the cloud and deploying at the edge,” said Chief Executive Officer Orr Danon. “There is no better place to do it because that’s where all the data comes together.”
Cloud providers know this and are doubling down on offerings focused on model training. Google is reportedly working on at least 21 different generative AI capabilities along with a portfolio of large language models. In June it released more than 60 generative AI models in its Vertex AI suite of cloud services. The search giant has made more than a dozen AI-related announcements this year.
“In most cases, with its strong third-party marketplace and ecosystem, Google Cloud can provide complete soup-to-nuts edge solutions with strong performance,” Sachin Gupta, vice president and general manager of the infrastructure and solutions group at the search giant, said in written remarks. “Google is able to provide a complete portfolio for inferencing, in our public regions or at the edge with Google Distributed Cloud.”
Introduced in 2021, Google Distributed Cloud brings Google’s cloud stack into customers’ data centers, allowing them to run on-premises applications with the same application programming interfaces, control planes, hardware and tools they use to connect with their other Google apps and services. Given the demand for AI services at the edge, it’s a good bet that the company could announce some additional AI services for Google Distributed Cloud at its Google Cloud Next conference this week in San Francisco.
AWS devoted much of its AWS Summit New York in July to discussing its AI plans and the theme is expected to dominate its giant re:Invent conference starting in late November. The company has been the most aggressive of the three big cloud providers in going after edge use cases.
“Customers are looking for solutions that provide the same experience from the cloud to on-premises and to edge applications,” an AWS spokesman said in written comments. “Regardless of where their applications may need to reside, customers want to use the same infrastructure, services, application program interfaces and tools.”
In addition to its on-premises cloud called Outposts, AWS has the Wavelength hardware and software stack that it deploys in carriers’ data centers. “Customers can use AWS Wavelength to perform low-latency operations and processing right where their data is generated,” the AWS spokesman said.
AWS’ IoT Greengrass ML Inference is a device that runs machine learning models trained in the cloud on local hardware. Even Snowball Edge, a device originally intended to be used to move large volumes of data to the cloud, has been outfitted to run machine learning.
Microsoft rolled out AI-optimized Azure instances earlier this year and invested $10 billion in OpenAI LLC. It has been the least active on the hardware front, although it’s reported to be close to announcing its own AI chipset. The company also offers Azure IoT Edge, which enables models trained in the cloud to be deployed at the edge. Microsoft declined to comment for this story.
Google’s Gupta says the search giant can provide a complete portfolio for inferencing in the cloud and at the edge. Photo: SiliconANGLE
Oracle Corp.’s Roving Edge Infrastructure, a ruggedized, portable and scalable cloud-compatible server node that the company introduced in 2021, has since been outfitted with graphics processing units for processing of AI workloads without network connectivity. Oracle declined to comment for this article.
Does any one provider have an edge? Probably not for long, said Matteo Gallina, principal consultant with global technology research and advisory firm Information Services Group Inc.
“One of them could have an initial breakthrough in certain capabilities but very soon, the others will follow,” he said in written comments. “The only exceptions are companies are strongly focused on the online gaming industry, which requires real-time rendering. They might have a foundational advantage.”
One wild card in the cloud giants’ edge strategies is microprocessors. With the GPUs that drive model training being costly and in short supply, all three providers have released or plan to release their own AI-optimized chipsets. Whether that silicon will ultimately make it into edge devices is still unclear, however.
Google Tensor Processing Unit AI training and inferencing chips have been around since 2015 but have so far only been used in the search giant’s cloud. Google relies primarily on partners for processing at the edge. “The ecosystem is critical so we can help solve problems completely rather than just provide an IaaS or PaaS layer,” Gupta said.
AWS has the Trainium chipset for training models and the Inferentia processor for running inferences but, like Google, has so far limited their use to its cloud. Microsoft’s plans for its still-unannounced AI chipset are unknown.
Experts say the big cloud providers are unlikely to be significant players in the fragmented and competitive market for intelligent edge devices. “The edge systems vendor will most likely be independent companies,” Linthicum said. “They will sell computing and storage at the edge to provide AI processing and model training, but a public cloud provider will control much of this centrally.”
“The hardware market is crowded and has many well-funded players,” said Gleb Budman, CEO of cloud storage provider Backblaze Inc. “Dozens of startups are targeting that market as are well-established companies like Intel and Nvidia.”
Ampere’s Wittich: “Inferencing is an area where public cloud providers have a compelling story.” Photo: X (Twitter)
Gartner’s Lowery noted that hyperscalers “are having trouble building silicon at scale” and that their on-premises cloud offerings – Outposts, Microsoft’s Stack Hub and Google’s Anthos and Distributed Cloud — “haven’t been wildly successful. There are a lot of players that are in a better position at the edge than the big cloud providers,” he said, “but there’s so much unmet opportunity that edge solutions might be secondary right now.”
But Jeff Wittich, chief product officer at edge processor maker Ampere Computing LLC, says the hyperscalers shouldn’t be counted out when it comes to low-latency workloads. Although they’re unlikely to enter the device market, they can leverage their points of presence to capture much of that business.
“Inferencing is an area where public cloud providers have a compelling story,” he said. “They can provide local access to all geographies around the world. The locality is well-suited.”
Evolving AI workloads and infrastructure are also opening up new possibilities on edge devices, said Jason McGee, chief technology officer of IBM Cloud. “I think the cloud operating model will apply across the spectrum,” he said. “The specific infrastructure platforms will evolve. We’re seeing a rise of GPUs but also how to run AI models on traditional CPUs.”
Deloitte’s Linthicum doesn’t expect many cloud computing executives to fret over a tiny hardware market share. “Public cloud providers will dominate the centralized processing for AI at the edge,” he said. “While they won’t own the edge device market, they will have systems that will allow for the development, deployment and operations of these devices, which is actually a much harder problem to solve.”
The bottom line, as he wrote recently in InfoWorld, is that “edge computing drives cloud computing.”
THANK YOU