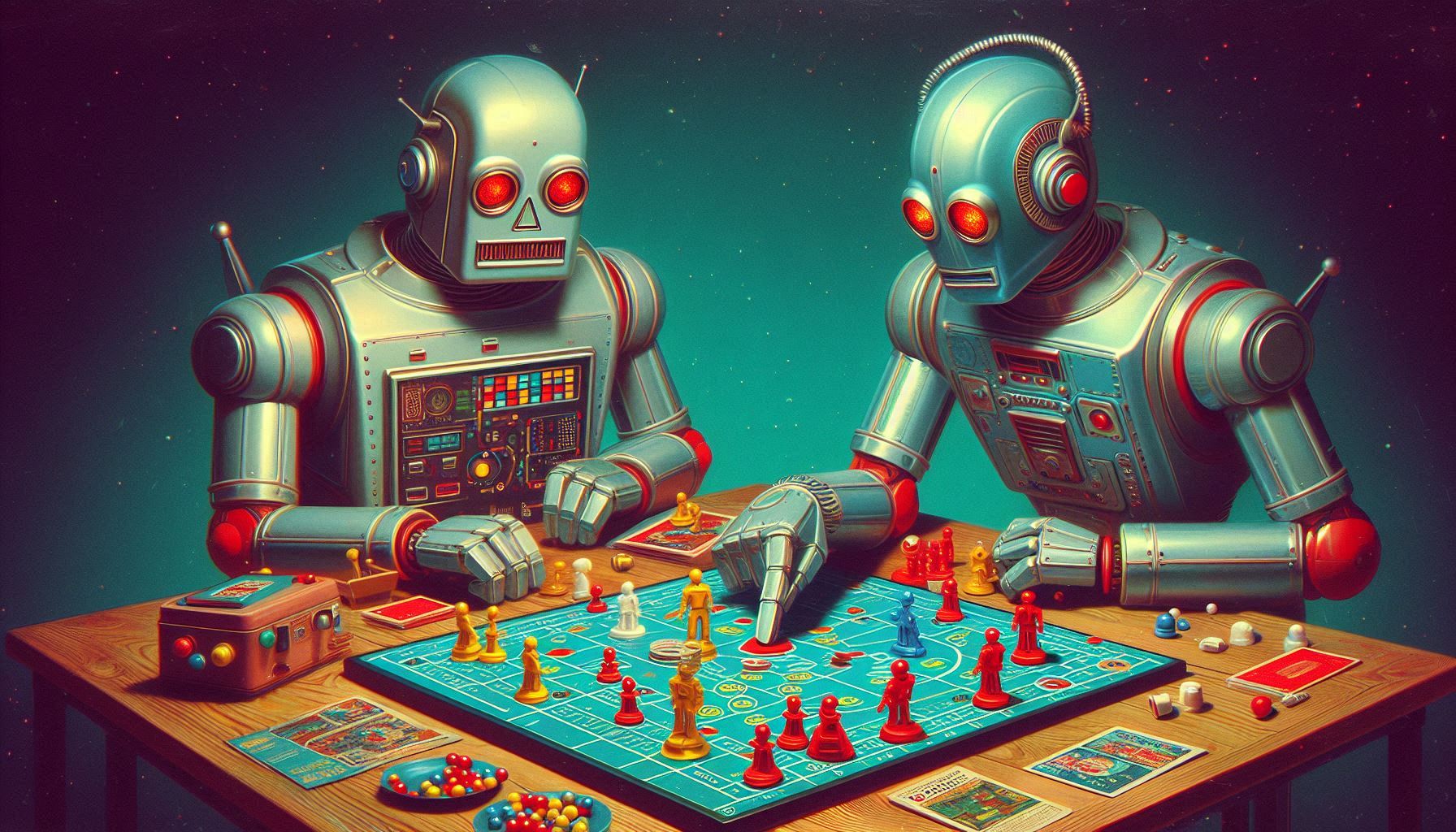
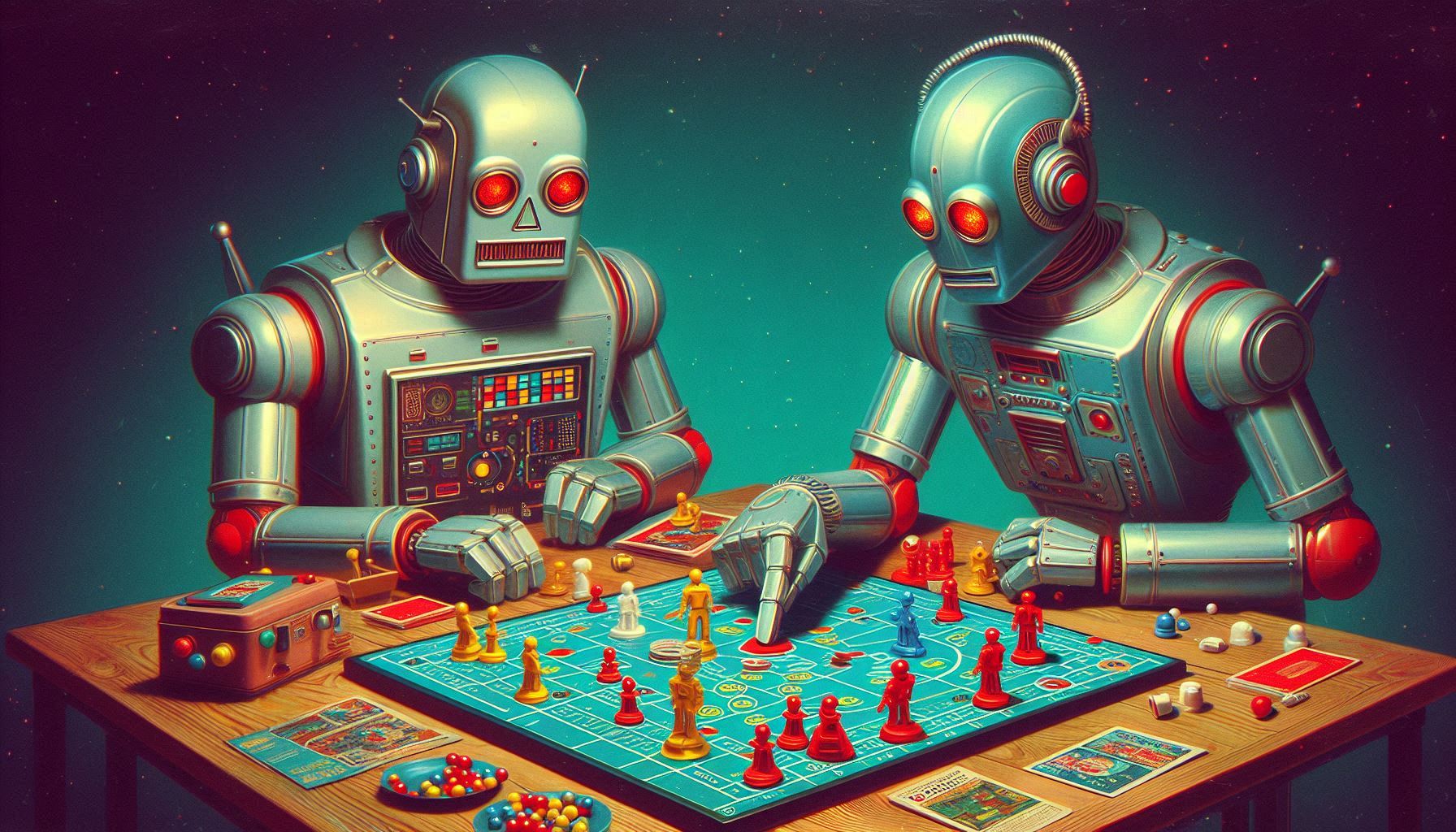
OpenAI today published a research paper that outlines a new way to improve the clarity and explainability of responses from generative artificial intelligence models.
The approach is designed to improve confidence in AI systems so they can be relied upon in the most critical use cases where accuracy is paramount. The method involves pitting two models against each other in a “prover-verifier game.” In this game, one model is assigned the role of “prover” and has to explain its solutions to a given problem, while the other is assigned as the “verifier” and is tasked with showing if the prover is right or wrong.
The goal of this game is to teach the prover to produce responses that are simple enough for the verifier to understand. According to OpenAI, this will result in AI models being able to deliver more comprehensible and verifiable outputs for human users.
In the study, OpenAI pitted a stronger GPT-4 model as the prover, against a much weaker version that played the role of verifier. The prover was told to alternate between being “helpful” or “sneaky” in different rounds, in order to throw off the verifier.
When set to be helpful, the prover would be rewarded for creating correct responses that the verifier liked and confirmed were sound. When it was set to be sneaky, it would be rewarded for generating an incorrect response that was able to fool the verifier.
Early on in the tests, the sneaky prover was easily able to trick the verifier with wrong answers. But over the course of the back-and-forth, the verifier, which was essentially being trained on each interaction, learned how to spot some of the subtle mistakes in the prover’s responses.
In response, the sneaky prover began pushing harder, using more advanced tactics and trickery to try and fool the verifier. The training ends when the prover is no longer able to fool the verifier.
For their experiments, OpenAI’s researchers tested the method using elementary school math problems. They discovered that the prover model that was optimized for correctness produced accurate but difficult-to-understand responses. They also learned that the verifier model was twice as effective as humans in trying to spot incorrect responses produced by the sneaky prover model.
The Prover-Verifier model gave more accurate and understandable results than a regular GPT-4. |
As the game progressed, the researchers found that the responses of the helpful prover models became increasingly easier to understand.
They concluded that a tough verifier model, which can reliably tell between right and wrong answers even when the prover is trying to trick it, combined with a helpful prover model, can be a powerful tool in the pursuit of AI explainability. It’s a promising way to build AI systems in industries where their responses need to be extremely trustworthy and accurate, such as in medicine, finance and law, OpenAI said.
According to the researchers, the main advantage of this method is that it’s much less reliant on human guidance and evaluations than other explainability solutions, meaning it can eliminate the need for direct human oversight.
THANK YOU